Keynote Speakers
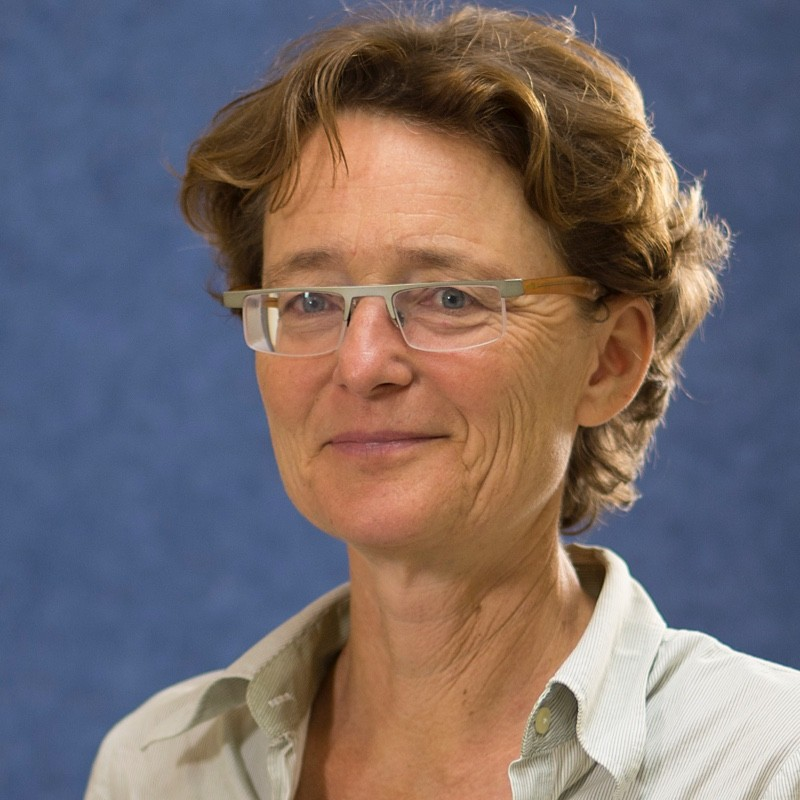
Prof. Dr. Ilse Aben is a Senior Scientist at SRON (Netherlands Institute for Space Research), Endowed Professor at Vrije Universiteit Amsterdam, and Co-Principal Investigator for TROPOMI (Tropospheric Monitoring Instrument onboard Sentinel-5P). Her expertise is in satellite remote sensing focusing on long lived greenhouse gases methane and carbon dioxide, and related species such as carbon monoxide. Her interest lies in measuring and understanding anthropogenic (and natural) emissions.
KEYNOTE:
"Using Satellites (and ML) to Support Governments and Companies to Reduce Methane Emissions."
The importance of reducing methane emissions to mitigate climate change in the short term has been recognised at the highest political level. At COP26 over 100 countries signed up to the Methane Pledge to reduce methane emissions by 30% by 2030. The International Methane Emission Observatory (IMEO) was recently launched by the UN to support companies and governments in reducing their methane emissions by providing actionable data based on a.o. methane measurements. In addition, the European Commission has prominently identified the role of satellites to support its goal to reduce methane emissions. In this presentation I will present the state-of-the-art on satellite observations of so-called methane super emitters, and how this can help reduce the emissions of this important greenhouse gas.
Dr. Michael F. Goodchild is Emeritus Professor of Geography at the University of California, Santa Barbara, where he also holds the title of Research Professor. He is also Distinguished Chair Professor at the Hong Kong Polytechnic University and Research Professor at Arizona State University, and holds many other affiliate, adjunct, and honorary positions at universities around the world. His research and teaching interests focus on issues in geographic information science, including uncertainty in geographic information, discrete global grids, and volunteered geographic information. He directed or co-directed several large funded projects, including the National Center for Geographic Information and Analysis, the Alexandria Digital Library, and the Center for Spatially Integrated Social Science.
KEYNOTE:
"Replicability Over The Globe"
Replicability takes on special meaning when researching phenomena that are embedded in space and time, including phenomena distributed on the surface and near-surface of the Earth. Two principles, spatial dependence and spatial heterogeneity, are generally characteristic of such phenomena. Various practices have evolved in dealing with spatial heterogeneity, including the use of place-based models. I review the rapidly emerging applications of artificial intelligence to phenomena distributed in space and time, and speculate on how the principle of spatial heterogeneity might be addressed. I introduce a concept of weak replicability and discuss possible approaches to its measurement.
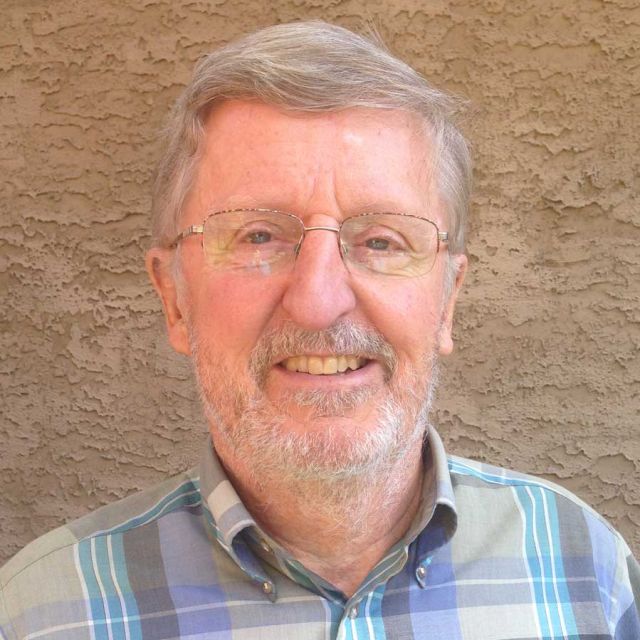
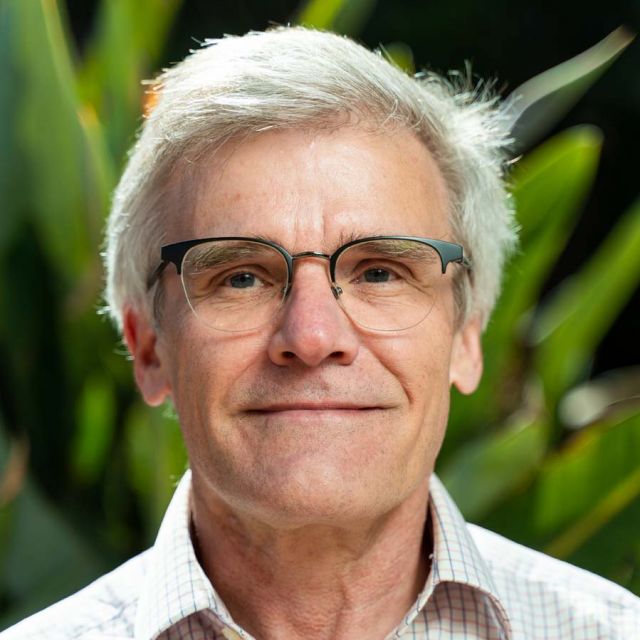
Dr. Werner Kuhn is a co-founder of the COSIT conference series and a member of several editorial boards of peer reviewed international journals. He is a professor of GIS at the University of California, Santa Barbara, USA and served as an assistant professor in the Department of Geoinformation at the Technical University Vienna in Austria before he became a post-doctoral researcher at the US National Center for Geographic Information and Analysis (NCGIA) at the University of Maine, USA. Also, he acquired his doctorate in 1989 from ETH Zurich, Switzerland. Dr. Werner Kuhn was also the Director of the UCSB Center for Spatial Studies and is one of the founding members of the Vespucci Initiative for Advancing Geographic Information Science, and a member of the Council of AGILE (Association of Geographic Information Laboratories in Europe, from 1998 to 2002), the international member of the Research Management Committee of the Canadian GEOIDE network (from 2001 to 2003), the Technical Director Europe of the Open GIS Consortium (from 1998 to 2001), and an Austrian delegate to CEN TC 287 on Geographic Information (from 1992 to 1995).
KEYNOTE:
"What you may wish you knew when you learned to use GIS"
Over the past decade, I have been trying to identify, understand, and specify a small set of Core Concepts of Spatial Information. These concepts are intended to be necessary and sufficient for understanding how spatial information answers questions about the world. I started researching them around 2010, when I faced two problems—and embarrassments—at once: First, I could not come up with contents that seemed compelling and worth teaching in a graduate course on Geographic Information Science. Second, I found it much harder than expected to help an epidemiologist improve his GIS model of how bird flu spreads among turkey farms in northern Italy. Both problems indicated to me a need for clarifying and strengthening the conceptual foundations of GIS. In my presentation at GeoMundus, I will summarize the research results so far and suggest that the core concepts have the potential to make learning and using GIS easier, more platform-independent, and more generally accessible. Through their systematic anchoring in physics and ontology (without compromises or “adaptations” to supposedly special needs of GIS) they can contribute to a future where spatial information and spatial computing are as well understood and applicable across disciplines as statistical information and computing are today.
Dr. Iris Kramer is the Founder & CEO of ArchAI, she is an archaeologist turned computer scientist, she is the first person in the world to do a PhD in deep learning for the detection of archaeological sites on Earth Observation data. ArchAI prides herself in the automation and assessment of archaeological sites required by environmental impact assessments (EIAs) during the planning permission application process for construction projects within Europe. Their pre-trained AI models can assess thousands of images instantly, providing rapid, accurate feedback to developers and project planners.
KEYNOTE:
"Using Deep Learning for Archaeological Site Discovery on Earth Observation Data"
Deep learning for automated detection of archaeological sites (objects) on earth observation data is a highly novel field. The key challenge of this field is in the inherent nature of the objects; they occur in small numbers, are sparsely located, and feature a unique pattern on the different remote sensing data modalities. I will introduce how we have alleviated these challenges by using specialised Convolutional Neural Networks (CNNs) and optimising training regimes. We quickly iterate through optimisations in small areas and work closely with domain experts to remove common false positives and thus reach a high level of accuracy before scaling the solution to large, in some cases national, datasets.
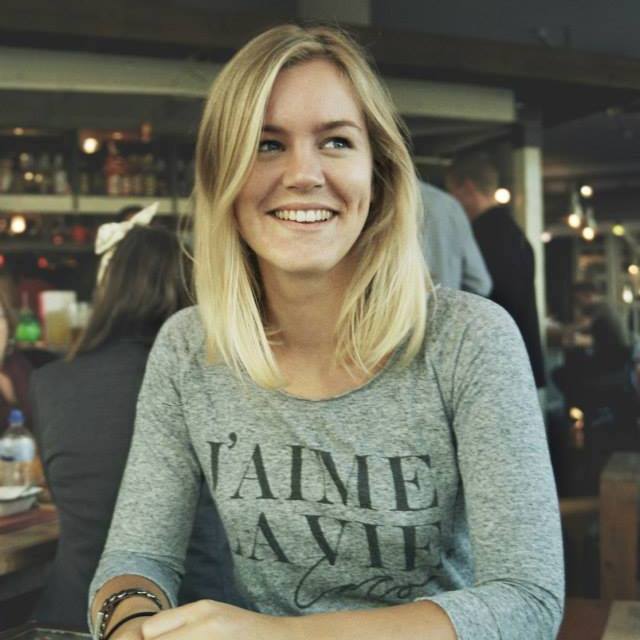